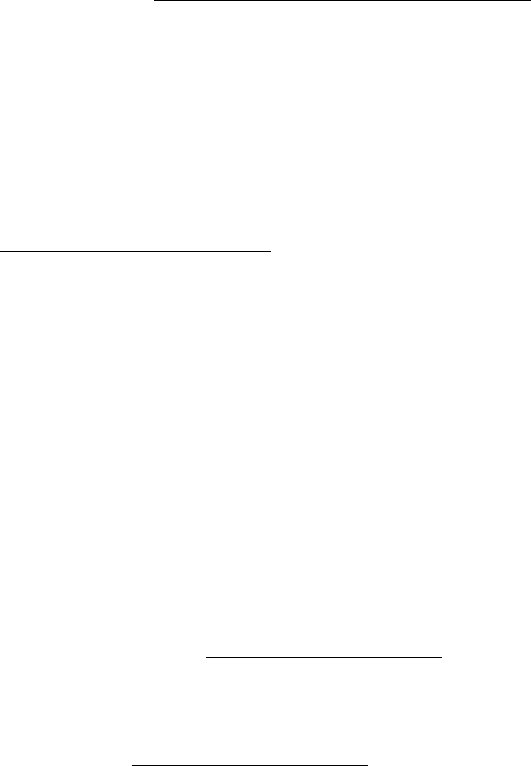
[54] Gine, Xavier, and Dean Karlan (2010). “Group versus Individual Liability: Long Term Evidence from
Philippine Microcredit Lending Groups.” Working Paper, Yale University (May).
[55] Hartman, Erin, Richard Grieve, Roland Ramsahai, and Jasjeet Sekhon (2013). “From SATE to PATT:
Combining Experimental with Observational Studies to Estimate Population Treatment Effects.”
Working Paper, University of California at Berkeley (October).
[56] Heck, Stefan, and Humayun Tai (2013). “Sizing the Potential of Behavioral Energy-Efficiency Initia-
tives in the US Residential Market.” White Paper, McKinsey & Company.
[57] Heckman, James (1979). “Sample Selection Bias as a Specification Error.” Econometrica, Vol. 47, No.
1 (January), pages 153-161.
[58] Heckman, James (1992). “Randomization and social policy evaluation.” In Charles Manski and Irwin
Garfinkel (Eds.), Evaluating Welfare and Training Programs. Harvard Univ. Press: Cambridge, MA,
pages 201-230.
[59] Heckman, James, Hidehiko Ichimura, Jeffrey Smith, and Petra Todd (1998). “Characterizing Selection
Bias Using Experimental Data.” Econometrica, Vol. 66, No. 5 (September), pages 1017-1098.
[60] Heckman, James, Hidehiko Ichimura, and Petra Todd (1997). “Matching as an Econometric Evaluation
Estimator: Evidence from Evaluating a Job Training Program.” Review of Economic Studies, Vol. 64,
No. 4, (October), pages 605-654.
[61] Heckman, James, Robert Lalonde, and Jeffrey Smith (1999). “The Economics and Econo-
metrics of Active Labor Market Programs.” In Orley Ashenfelter and David Card (Eds.),
Handbook of Labor Economics, Chapter 31, pages 1865-2097.
[62] Heckman, James, and Jeffrey Smith (1995). “Assessing the Case for Social Experiments.” Journal of
Economic Perspectives, Vol. 9, No. 2 (Spring), pages 85-110.
[63] Heckman, James, and Jeffrey Smith (1997). “The Sensitivity of Experimental Impact Estimates: Evi-
dence from the National JTPA Study,” NBER Working Paper No. 6105 (July).
[64] Heckman, James, Sergio Urzua, and Edward Vytlacil (2006). “Understanding Instrumental Variables
in Models with Essential Heterogeneity.” The Review of Economics and Statistics, Vol. 88, No. 3
(August), pages 389-432.
[65] Heckman, James, and Edward Vytlacil (2001). “Policy-Relevant Treatment Effects.” American Eco-
nomic Review, Vol. 91, No. 2 (May), pages 107-111.
[66] Heckman, James, and Edward Vytlacil (2005). “Structural Equations, Treatment Effects, and Econo-
metric Policy Evaluation.” Econometrica, Vol. 73, No. 3 (May), pages 669–738.
[67] Heckman, James, and Edward Vytlacil (2007a). “Econometric Evaluation of Social Programs, Part
I: Causal Models, Structural Models and Econometric Policy Evaluation.” In James Heckman and
Edward Leamer (Eds), Handbook of Econometrics, Vol. 6B. Amsterdam: Elsevier, pages 4779-4874.
[68] Heckman, James, and Edward Vytlacil (2007b). “Econometric Evaluation of Social Programs, Part
II: Using the Marginal Treatment Effect to Organize Alternative Econometric Estimators to Evaluate
Social Programs, and to Forecast their Effects in New Environments.” In James Heckman and Edward
Leamer (Eds), Handbook of Econometrics, Vol. 6B. Amsterdam: Elsevier, pages 4875-5144.
[69] Higgins, Julian, and Simon Thompson (2002). “Quantifying Heterogeneity in a Meta-Analysis.” Statis-
tics in Medicine, Vol. 21, No. 11 (June 15), pages 1539-1558.
[70] Hotz, Joseph (1992). “Designing Experimental Evaluations of Social Programs: The Case of the U.S.
National JTPA Study.” University of Chicago Harris School of Public Policy Working Paper 9203
(January).
40